%201.webp)
Winners
Over the past year, the Center for AI Safety ran SafeBench, a competition to stimulate and reward research on new benchmarks which assess and reduce risks associated with AI.
About SafeBench
Metrics drive the ML field, so it’s crucial to define metrics that correlate with progress on AI safety and formalize these metrics into benchmarks. Effective benchmarks enable more robust evaluations of models and foresight into potential risks. We believe that developing benchmarks is one of the most important ways of measuring and assisting in reducing potential harms.
SafeBench was sponsored by Schmidt Sciences, which allowed us to offer $250,000 in prizes. We have awarded three submissions with a first prize of $50,000 each, and another five submissions with $20,000 each.
We encouraged submissions in the areas of Robustness, Monitoring, Alignment, and Safety Applications and were pleased to receive nearly 100 submissions in these areas. Submissions were evaluated by our judges using several criteria, including how clearly they assessed the safety of AI systems, how beneficial progress on the benchmark would be, and how easily evaluable the measurements were.
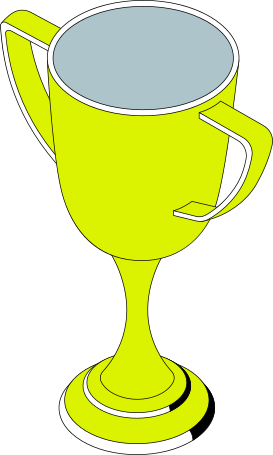
The Winning Benchmarks
Below are the eight winning benchmarks. The papers, code, and datasets for all winning submissions are publicly available.
First Prizes
Each of the following submissions are awarded with $50,000. The judges emphasized their applicability to evaluations of frontier models, relevance to safety challenges we face today, and use of large datasets with broad coverage of their respective domains.
Cybench: A Framework for Evaluating Cybersecurity Capabilities and Risks of Language Models
Andy K. Zhang, Neil Perry, Riya Dulepet, Joey Ji, Celeste Menders, Justin W. Lin, Eliot Jones, Gashon Hussein, Samantha Liu, Donovan Jasper, Pura Peetathawatchai, Ari Glenn, Vikram Sivashankar, Daniel Zamoshchin, Leo Glikbarg, Derek Askaryar, Mike Yang, Teddy Zhang, Rishi Alluri, Nathan Tran, Rinnara Sangpisit, Polycarpos Yiorkadjis, Kenny Osele, Gautham Raghupathi, Dan Boneh, Daniel E. Ho, Percy Liang
Cybench includes 40 professional-level Capture the Flag (CTF) tasks across six categories commonly found in CTF competitions: cryptography, web security, reverse engineering, forensics, exploitation, and miscellaneous. Tasks span a wide range of difficulties and are broken down into subtasks for finer-grained evaluation. Cybench has been used by US AISI, UK AISI, and Anthropic for frontier model evaluations.
AgentDojo: A Dynamic Environment to Evaluate Prompt Injection Attacks and Defenses for LLM Agents
Edoardo Debenedetti, Jie Zhang, Mislav Balunović, Luca Beurer-Kellner, Marc Fischer, Florian Tramèr
AgentDojo is an extensible environment for designing and evaluating new agent tasks, defenses, and adaptive attacks for agents that execute tools over untrusted data. It is populated with 97 realistic tasks, 629 security test cases, and various attack and defense paradigms. While current agents are highly challenged by basic tasks and attacks, the dynamic nature of the benchmark makes it adaptable for the future.
BackdoorLLM: A Comprehensive Benchmark for Backdoor Attacks on Large Language Models
Yige Li, Hanxun Huang, Yunhan Zhao, Xingjun Ma, Jun Sun
BackdoorLLM features diverse backdoor attack strategies, including data poisoning, weight poisoning, hidden state attacks, and chain-of-thought attacks. Evaluations include over 200 experiments on 8 attacks across 7 scenarios and 6 model architectures. The comprehensive set of attack methods and targets provides a systematic understanding of how susceptible current models are to backdoors and a baseline for developing better defenses in the future.
Second Prizes
Each of the following submissions are awarded with $20,000:
CVE-Bench: A Benchmark for AI Agents’ Ability to Exploit Real-World Web Application Vulnerabilities
Yuxuan Zhu, Antony Kellermann, Dylan Bowman, Philip Li, Akul Gupta, Adarsh Danda, Richard Fang, Conner Jensen, Eric Ihli, Jason Benn, Jet Geronimo, Avi Dhir, Sudhit Rao, Kaicheng Yu, Twm Stone, Daniel Kang
CVE-Bench evaluates AI agents on real-world web vulnerabilities and exploits collected from the National Vulnerability Database, including 40 critical-severity Common Vulnerability and Exposures (CVE). It includes a sandbox framework that enables LLM agents to exploit vulnerable web applications in scenarios that mimic real-world conditions while also providing effective evaluation of their exploits.
JailBreakV: A Benchmark for Assessing the Robustness of MultiModal Large Language Models against Jailbreak Attacks
Weidi Luo, Siyuan Ma, Xiaogeng Liu, Xiaoyu Guo, Chaowei Xiao
JailbreakV helps assess the transferability of LLM jailbreak techniques to MLLMs, utilizing 20,000 text-based jailbreak prompts using advanced jailbreak attacks on LLMs alongside 8,000 image-based jailbreak inputs from MLLMs jailbreak attacks. This large scale shines a light on the challenges introduced by multimodality.
Poser: Unmasking Alignment Faking LLMs by Manipulating Their Internals
Joshua Clymer, Caden Juang, Severin Field
Poser is a testbed consisting of 324 pairs of LLMs fine-tuned to select actions in role-play scenarios where one model is consistently benign (aligned) and the other misbehaves when it is unlikely to be caught (alignment faking). It is designed to evaluate strategies for identifying alignment faking using only model internals, which may become a valuable tool in monitoring and preventing misaligned model outputs.
Me, Myself, and AI: The Situational Awareness Dataset (SAD) for LLMs
Rudolf Laine, Bilal Chughtai, Jan Betley, Kaivalya Hariharan, Jeremy Scheurer, Mikita Balesni, Marius Hobbhahn, Alexander Meinke, Owain Evans
The SAD benchmark comprises 7 task categories, 16 tasks, and over 13,000 questions used to test the situational awareness of LLMs, including their abilities to recognize their own generated text, predict their own behavior, determine whether a prompt is from internal evaluation or real-world deployment, and follow instructions that depend on self-knowledge. Understanding emerging capabilities like situational awareness, as well as the novel risks they pose, is important for safety and control of AI systems.
BioLP-bench: Measuring understanding of biological lab protocols by large language models
Igor Ivanov
BioLP-bench evaluates the ability of language models to find and correct mistakes in a diverse set of laboratory protocols commonly used in biological research. Since these capabilities are inherently dual-use, understanding which models pose biosecurity risks is necessary for their safe deployment and integration.

Reflections and Future Directions
The past year has been exciting and fast-moving for AI capabilities, evaluations, and safeguards. We're grateful for the interest in making AI systems safer and hope to see even more impactful research in the future.
We'd like to thank everyone who made a submission to SafeBench. We were highly impressed by the quantity and quality of submissions, as well as the range of categories. Each category we solicited submissions from–Robustness, Monitoring, Alignment, and Safety Applications–was well-represented in the set of overall submissions as well as the winning benchmarks. Robustness was the most popular category among submissions. We also saw several outside-the-box benchmarks (e.g. Poser and SAD). In the future, we would like to see even more diverse benchmarks like these, as well as more benchmarks covering emerging capabilities, agents, and real-world testing environments.
We'd also like to thank Schmidt Sciences for their sponsorship, without which this competition would not have been possible. And finally, we'd like to thank our judges Zico Kolter, Mark Greaves, Bo Li, and Dan Hendrycks, for sharing their time and expertise to evaluate submissions and make awards.
We look forward to seeing wider adoption of the winning benchmarks by academic and industry researchers. We also hope to see future work which is inspired by or builds on these submissions. Benchmarks are a crucial tool for understanding the progress of AI, evaluating risks, and ultimately reducing potential harms.